Machine Learning Aims to Ease Identification of Sustainable Composite Materials
A new model could help engineers reduce plastic use and adopt biomass or renewable materials through a rapid search for sustainable composite alternatives.
June 1, 2022
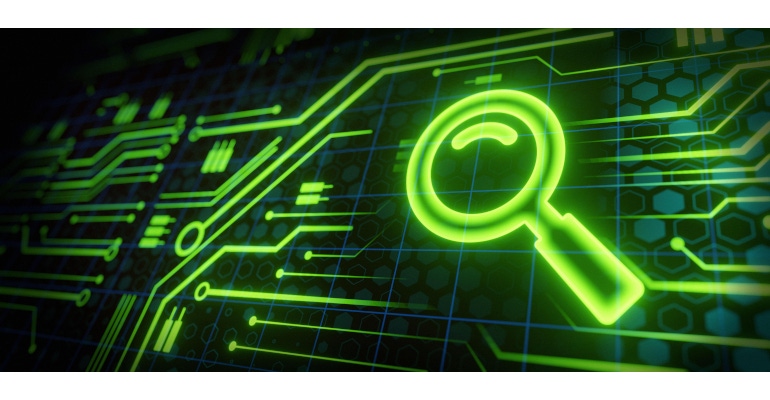
The saying that there is strength in numbers can apply to materials as well. Two or more materials make up what are called composites and together their performance can greatly exceed that of single materials.
Some of these materials may not be sustainable, though, so many companies are looking for substitute composite materials. But these substitutions may reduce performance compared with that of the original material, not only due to the features of the individual constituent materials, such as their physicochemical properties, but also because of the interactions between the constituents.
“Finding a new composite material that achieves the same performance as the original using human experience and intuition alone takes a very long time because you have to evaluate countless materials while also taking into account the interactions between them,” explained Michihiro Okuyama, assistant manager at Konica Minolta Inc., in a company statement.
To speed up this process, researchers from Konica Minolta and the Nara Institute of Science and Technology in Japan have developed a machine learning method that conducts rapid searches among many materials, based on the relationship between the materials’ features and performance.
When the properties of the constituent materials are unknown, predictive searches are difficult. To overcome this limitation, the researchers developed a new type of machine learning method for finding alternative materials. The new method can quantitatively evaluate the interactions among the component materials to reveal how much they contribute to the overall performance of the composite. The method then searches for replacement constituents with similar performance to the original material.
The researchers tested their method by searching for alternative constituent materials for a composite consisting of three materials—resin, a filler, and an additive. They experimentally evaluated the performance of the substitute materials identified by machine learning and found that they were similar to the original material, proving that the model works. Their work was published in the journal Science and Technology of Advanced Materials: Methods.
“In developing alternatives that make up composite materials, our new machine learning method removes the need to test large numbers of candidates by trial and error, saving both time and money,” concluded Okuyama.
About the Author(s)
You May Also Like